It is been a long time since I’ve posted something here, roughly corresponding with the start of the COVID pandemic. During that time I didn’t travel as much and it was easy to not think about the value of communicating with the outside. In Fall 2019, I tore my quad (and broke my kneecap and toe) and this put me in less of a communicative mood. We also found out in Spring 2020 that my wife was pregnant. Delivering our first child, Ayla Helen Fuller on September 24, 2020.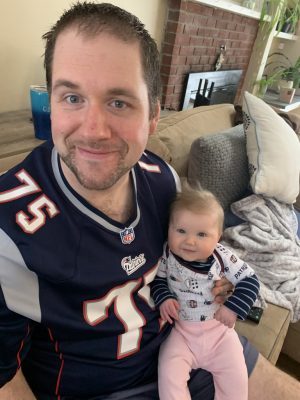
We were trying to be first time parents at home while preparing for tenure application. Honestly, the COVID pandemic was really hard on research productivity. It was particularly hard for theory students to make progress without in person white board sessions. It seemed that everyone had much more to do without making much progress.
I had a streak of about 10 straight rejections including a record of 8 submissions for a paper before acceptance. Coupled with several important grant rejections/cancellations this left the lab in a rough place. Thanks to some help from UConn we’ve managed to keep supporting students. It is been a tough two years.
I’m writing because in academia (and all aspects of life) we see others success and tend to see people that are vastly more successful/capable than we are and compare to them. Everyone sees the professor/Ph.D. student that is publishing multiple times every year at the top conference. As they say “Comparison is the thief of joy.”
I’m writing this post because that I’ve submitted my tenure packet to UConn and from here on out (assuming I don’t lose my job) any comparison that I do will be purely internal. I’m writing this post in part to remind myself that all comparisons are my doing and they never make me feel good. I’m sure there’s some people reading this who view me as a competent member of a community, some who don’t know who am I, and some who aspire to the level of success I have achieved.
All I know is that I love teaching, mentoring students, discovering new results, and communicating science to others. I don’t know how good I am at any of these things. I’d like to say that I don’t care but I do deeply. Maybe that will change someday as external pressures reduce. But for now I’m looking back through my CV and seen I’ve:
- Co-authored papers with 56 people,
- Supervised 8 graduate students and learn a ton from all of them,
- Supervised 23 undergraduates in research in five years at UConn (including 8 honors thesis), and
- Built four new courses (Crypto II, Network Security, Computer Security, and our Cybersecurity Lab)
I’m going to try and measure my success in terms of positive impact made on those around me. I am thrilled to work with wonderful people and I hope I can help them achieve their dreams. I’m not the best in this field, I’m not the worst (either as a person or a researcher). I believe I’m helping the world and those around me.
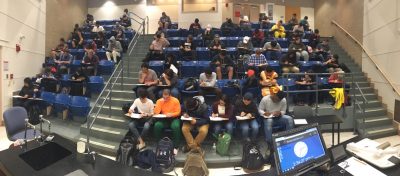
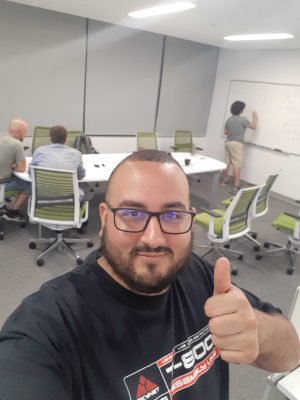